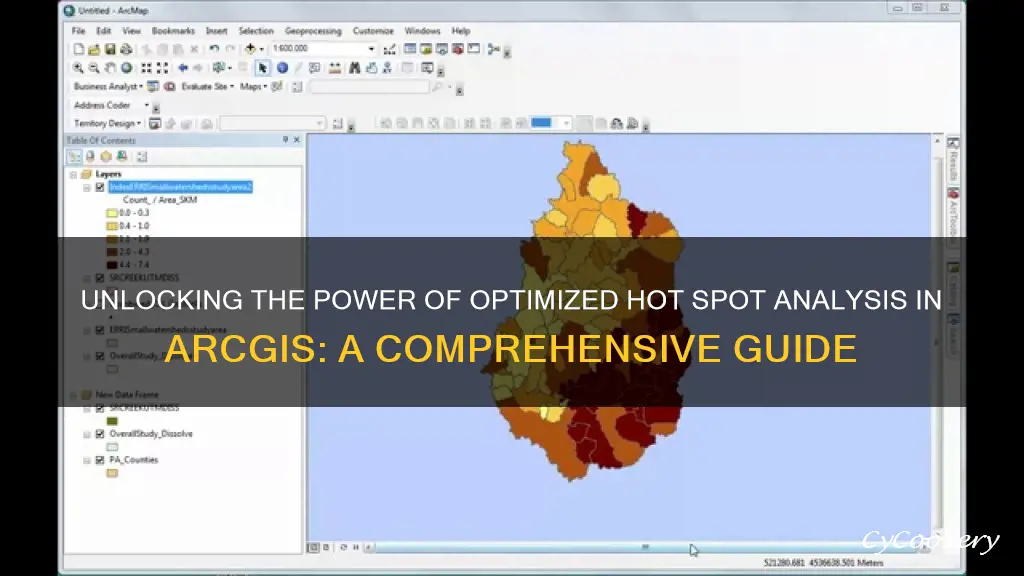
Optimized Hot Spot Analysis is a tool that identifies statistically significant spatial clusters of high values (hot spots) and low values (cold spots) using the Getis-Ord Gi* statistic. It evaluates the characteristics of the input feature class to produce optimal results. This tool is particularly useful for those who want to answer questions such as Where do high and low values cluster? and Where are there many points?.
Characteristics | Values |
---|---|
What it does | Identifies statistically significant spatial clusters of high values (hot spots) and low values (cold spots) |
Input | Incident points or weighted features (points or polygons) |
Output | A map of statistically significant hot and cold spots |
Output Feature Class | A z-score, p-value, and confidence level bin (Gi_Bin) for each feature in the Input Feature Class |
Incident data | Points representing events (e.g. crime, traffic accidents) or objects (e.g. trees, stores) |
Analysis Field | Counts (e.g. number of traffic accidents at street intersections), rates (e.g. city unemployment), averages (e.g. mean math test score among schools), indices (e.g. consumer satisfaction score for car dealerships) |
Incident Data Aggregation Method | Count incidents within fishnet grid, count incidents within hexagon grid, count incidents within aggregation polygons, snap nearby incidents to create weighted points |
Bounding Polygons Defining Where Incidents Are Possible | A polygon feature class defining where the incident Input Features could possibly occur |
Polygons For Aggregating Incidents Into Counts | The polygons used to aggregate the incident Input Features to get an incident count for each polygon feature |
Distance Band or Threshold Distance | Specifies a cutoff distance for the inverse distance and fixed distance options |
Weights Matrix File | A file containing weights that define spatial, and potentially temporal, relationships among features |
What You'll Learn
- Optimized Hot Spot Analysis identifies statistically significant spatial clusters of high values (hot spots) and low values (cold spots)
- It automatically aggregates incident data
- It identifies an appropriate scale of analysis
- It corrects for multiple testing and spatial dependence
- It creates a new Output Feature Class with a z-score, p-value and confidence level bin (Gi_Bin) for each feature in the Input Feature Class
Optimized Hot Spot Analysis identifies statistically significant spatial clusters of high values (hot spots) and low values (cold spots)
Optimized Hot Spot Analysis is a tool that identifies statistically significant spatial clusters of high values (hot spots) and low values (cold spots). It does so by evaluating the characteristics of the input feature class to produce optimal results. The tool is especially useful when you want to answer questions like "Where do high and low values cluster?" or "Where are there many points?".
The analysis field you select might represent counts (such as the number of traffic accidents at street intersections), rates (such as city unemployment, where each city is represented by a point feature), averages (such as the mean math test score among schools), or indices (such as a consumer satisfaction score for car dealerships across the country).
The Optimized Hot Spot Analysis tool automatically aggregates incident data, identifies an appropriate scale of analysis, and corrects for both multiple testing and spatial dependence. It interrogates your data to determine settings that will produce optimal hot spot analysis results. The computed settings used to produce these results are reported in the Results window.
This tool creates a new Output Feature Class with a z-score, p-value, and confidence level bin (Gi_Bin) for each feature in the Input Feature Class. The z-score and p-value fields are measures of statistical significance that indicate whether the observed spatial clustering of high or low values is more pronounced than one would expect in a random distribution of those same values. The Gi_Bin field identifies statistically significant hot and cold spots, corrected for multiple testing and spatial dependence using the False Discovery Rate (FDR) correction method.
If you want full control over these settings, you can use the Hot Spot Analysis tool instead.
Anodized Cookware: Safe or Not?
You may want to see also
It automatically aggregates incident data
ArcGIS Optimized Hot Spot Analysis is a tool that automatically aggregates incident data, identifies an appropriate scale of analysis, and corrects for multiple testing and spatial dependence. It is designed to determine the settings that will produce optimal hot spot analysis results.
The tool works by interrogating your data to obtain the settings that will yield the best results. It is similar to the way that the automatic setting on a digital camera uses lighting and subject versus ground readings to determine the optimal aperture, shutter speed, and focus.
Incident data refers to points representing events (e.g., crime, traffic accidents) or objects (e.g., trees, stores) where the focus is on the presence or absence rather than a measured attribute associated with each point.
Optimized Hot Spot Analysis automatically aggregates incident data by counting incidents within a defined area, such as a fishnet grid, hexagon grid, or aggregation polygons. This process involves collapsing coincident points into a single point at each unique location in the dataset and counting the number of incidents within each polygon cell or aggregation polygon.
The appropriate scale of analysis is identified by the tool using strategies such as Incremental Spatial Autocorrelation, which measures the intensity of spatial clustering at increasing distances. The ideal scale of analysis matches the scale of the question being asked. For example, if you are looking for hot spots of a disease outbreak and know that the mosquito vector has a range of 10 miles, using a 10-mile distance would be appropriate.
The output of the Optimized Hot Spot Analysis tool includes a new Output Feature Class with a z-score, p-value, and confidence level bin (Gi_Bin) for each feature in the Input Feature Class. The Gi_Bin field identifies statistically significant hot and cold spots, corrected for multiple testing and spatial dependence using the False Discovery Rate (FDR) correction method.
By automatically aggregating incident data and identifying the appropriate scale of analysis, the Optimized Hot Spot Analysis tool in ArcGIS provides valuable insights into spatial patterns and supports decision-making in various fields, including crime analysis, epidemiology, and traffic incident analysis.
The Quest for Cool: Unveiling the Mystery of Cold-Handled Cast Iron Pans
You may want to see also
It identifies an appropriate scale of analysis
Optimized Hot Spot Analysis is a tool that identifies statistically significant spatial clusters of high values (hot spots) and low values (cold spots). It does so by evaluating the characteristics of the input feature class to produce optimal results.
The tool interrogates the data to obtain the settings that will yield optimal hot spot results. It automatically aggregates incident data and identifies an appropriate scale of analysis. This is similar to the way that the automatic setting on a digital camera will use lighting and subject versus ground readings to determine an appropriate aperture, shutter speed, and focus.
The Optimized Hot Spot Analysis tool identifies an appropriate scale of analysis by using strategies such as Incremental Spatial Autocorrelation. This tool performs the Global Moran's I statistic method for a series of increasing distances, measuring the intensity of spatial clustering for each distance. The intensity of clustering is determined by the z-score returned. Typically, as the distance increases, so does the z-score, indicating intensification of clustering. At some particular distance, however, the z-score generally peaks. Peaks reflect distances where the spatial processes promoting clustering are most pronounced. The Optimized Hot Spot Analysis tool identifies peak distances using Incremental Spatial Autocorrelation. If a peak distance is found, this distance becomes the scale of analysis. If multiple peak distances are found, the first peak distance is selected.
When no peak distance is found, the Optimized Hot Spot Analysis tool examines the spatial distribution of the features and computes the average distance that would yield K neighbors for each feature. K is computed as 0.05 * N, where N is the number of features in the Input Features layer. K will be adjusted so it is never smaller than 3 or larger than 30. If the average distance that would yield K neighbors exceeds one standard distance, the scale of analysis will be set to one standard distance; otherwise, it will reflect the K neighbor average distance.
Incremental Spatial Autocorrelation can be time-consuming for large, dense datasets. Therefore, when a feature with 500 or more neighbors is encountered, the incremental analysis is skipped, and the average distance that would yield 30 neighbors is computed and used for the scale of analysis.
The distance reflecting the scale of analysis will be reported as messages during tool execution and will be used to perform the hot spot analysis. This distance corresponds to the Distance Band or Threshold Distance parameter used by the Hot Spot Analysis (Getis-Ord Gi*) tool.
Building a BBQ Hot Pot: A Step-by-Step Guide
You may want to see also
It corrects for multiple testing and spatial dependence
The Optimized Hot Spot Analysis tool identifies statistically significant spatial clusters of high values (hot spots) and low values (cold spots). It automatically aggregates incident data, identifies an appropriate scale of analysis, and corrects for both multiple testing and spatial dependence.
The tool interrogates your data to determine settings that will produce optimal hot spot analysis results. It uses the Getis-Ord Gi* statistic to create a map of statistically significant hot and cold spots. The Gi* statistic returned for each feature in the dataset is a z-score. The z-score and p-value fields do not reflect any kind of FDR (False Discovery Rate) correction.
The Gi_Bin field identifies statistically significant hot and cold spots, corrected for multiple testing and spatial dependence using the False Discovery Rate (FDR) correction method. Features in the +/-3 bins (features with a Gi_Bin value of either +3 or -3) are statistically significant at the 99% confidence level; features in the +/-2 bins reflect a 95% confidence level; features in the +/-1 bins reflect a 90% confidence level; and the clustering for features with 0 for the Gi_Bin field is not statistically significant.
The statistical significance reported in the Output Features is automatically adjusted for multiple testing and spatial dependence using the FDR correction method. The FDR correction is also applied to the z-scores, which indicate the intensity of clustering of high or low values.
The Optimized Hot Spot Analysis tool is particularly useful when you want to identify spatial clusters of high or low values without having full control over the settings. If you require full control over the settings, you can use the Hot Spot Analysis tool instead.
Stackable Pans: Instant Pot-Friendly?
You may want to see also
It creates a new Output Feature Class with a z-score, p-value and confidence level bin (Gi_Bin) for each feature in the Input Feature Class
ArcGIS Optimized Hot Spot Analysis is a tool that identifies statistically significant spatial clusters of high values (hot spots) and low values (cold spots). It does this by evaluating the characteristics of the input feature class to produce optimal results. The output of this tool is a new Output Feature Class with a z-score, p-value, and confidence level bin (Gi_Bin) for each feature in the Input Feature Class.
The z-score is a standard deviation, which indicates how far the result is from the average. In the context of spatial analysis, a very high or very low (negative) z-score, associated with a very small p-value, indicates that it is unlikely that the observed spatial pattern is due to random chance.
The p-value is a probability that the observed spatial pattern was created by some random process. A very small p-value means that it is very unlikely that the observed spatial pattern is the result of random processes, so the null hypothesis can be rejected.
The confidence level represents the degree of risk that you are willing to accept for being wrong (for falsely rejecting the null hypothesis). Typical confidence levels are 90%, 95%, or 99%. A 99% confidence level would indicate that you are only willing to reject the null hypothesis if the probability that the pattern was created by random chance is less than 1%.
The Gi_Bin field identifies statistically significant hot and cold spots, corrected for multiple testing and spatial dependence using the False Discovery Rate (FDR) correction method. The bins are assigned based on the confidence level, with features in the +/-3 bins being statistically significant at the 99% confidence level, +/-2 bins reflecting a 95% confidence level, and +/-1 bins reflecting a 90% confidence level.
By creating a new Output Feature Class with these values, the Optimized Hot Spot Analysis tool in ArcGIS provides a comprehensive set of statistics and visualisations to help users identify and understand spatial clusters in their data.
The Heat Within: Understanding Clay Flower Pots' Temperature Limits
You may want to see also
Frequently asked questions
Optimized Hot Spot Analysis is a tool that identifies statistically significant spatial clusters of high values (hot spots) and low values (cold spots) using the Getis-Ord Gi* statistic.
This tool creates a map of statistically significant hot and cold spots. It automatically aggregates incident data, identifies an appropriate scale of analysis, and corrects for both multiple testing and spatial dependence.
Incident data are points representing events (e.g. crime, traffic accidents) or objects (e.g. trees, stores) where your focus is on presence or absence rather than a measured attribute associated with each point.
The Getis-Ord Gi* statistic calculates a z-score for each feature in a dataset. The z-scores and p-values tell you where features with either high or low values cluster spatially.